Subjective-Objective-Assessment-Plan notes using Large Language Models
This project is to Identify key terms in history gathering and physical examination based on a standard format for structuring medical notes: Subjective-Objective-Assessment-Plan notes using Large Language Models.
Medical students need to practice writing notes documenting patient’s medical and health information and physician diagnostic reasoning. The assessment of the written notes (also called Subjective-Objective-Assessment-Plan–SOAP–notes) is tedious and expensive, requiring many hours of time from medical experts and affording limited and delayed learner feedback. Natural Language Processing (NLP) methods could automate the scoring process and provide immediate feedback to medical students. However, the significant effort required to create an annotated dataset increases the challenge of using traditional supervised NLP methods particularly when clinical cases change. Our goal is to develop NLP algorithms to grade SOAP notes with high accuracy and adaptability. The proposed pre-training, fine-tuning and in-context learning paradigms will establish a weakly supervised framework utilizing transfer learning for automatic assessment of SOAP notes with a low number of annotations needed.
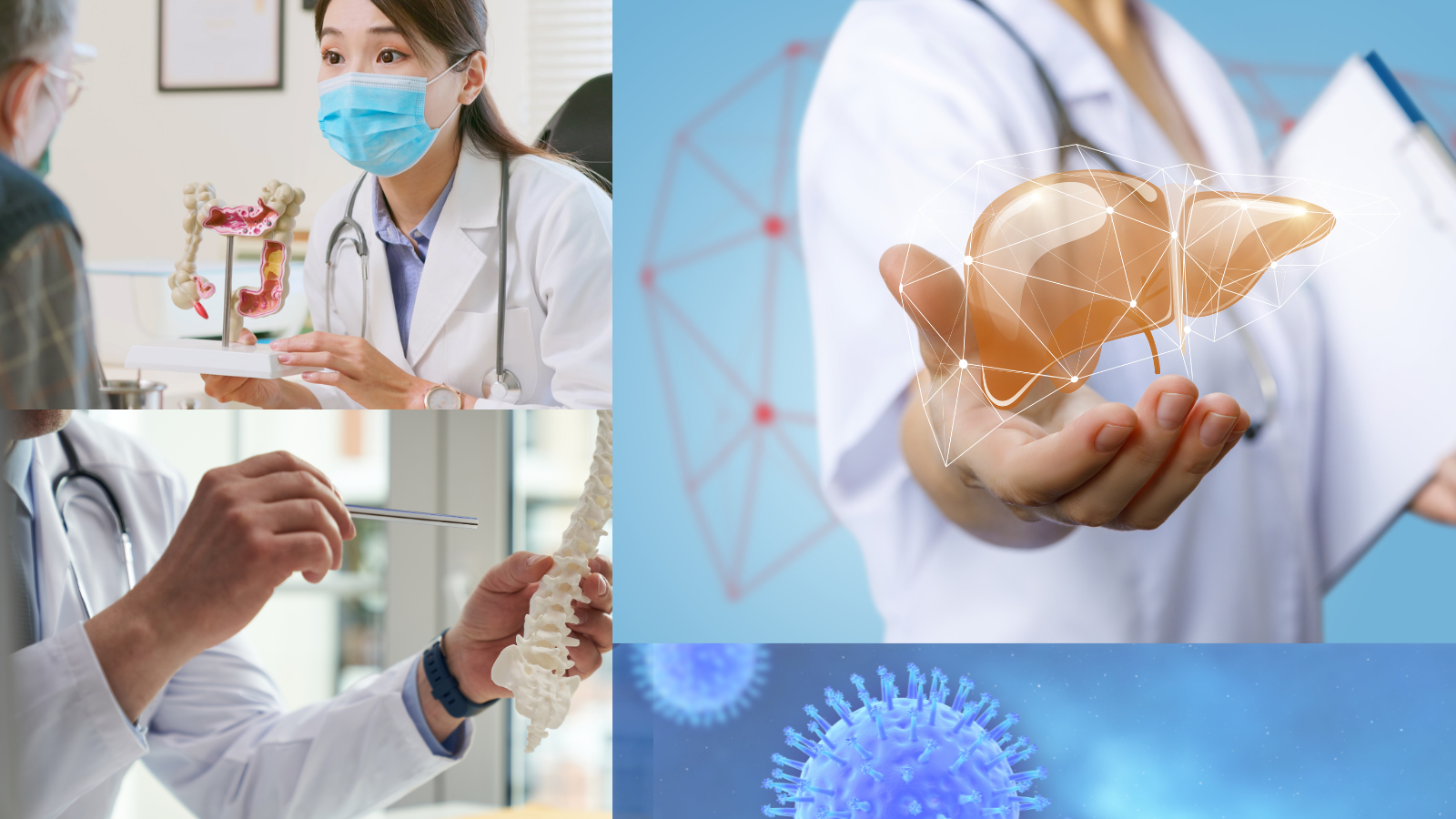